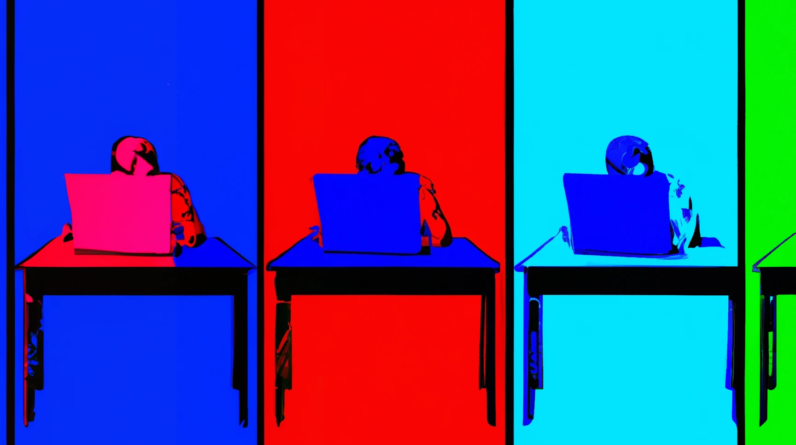
The language models behind ChatGPT and other generative AIs are trained on written words that have been taken from libraries, taken from websites and social networks, and taken from news reports and transcripts of speeches from around the world. There are 250 billion such words behind GPT-3.5, the model that powers ChatGPT, for example, and GPT-4 is already here.
now new research from Stanford University has quantified exactly how well (or, actually, poorly) these models align with the views of US demographics, showing that language models have a decided bias on hot topics that may be out of line with general popular sentiment.
“Some language models fail to capture the subtleties of human opinion and often simply express the dominant viewpoint of certain groups, while under-representing those of other demographic subgroups,” he says. Shibani Santurkar, former postdoctoral researcher at Stanford and first author of the study. “They should be more aligned.”
In the paper, a research team including Stanford postdoctoral fellow Esin Durmus, Columbia doctoral student Faisal Ladhak, Stanford doctoral student Cinoo Lee, and Stanford computer science professors Percy Liang i Tatsunori Hashimoto presents OpinionQA, a tool for assessing bias in linguistic models. OpinionQA compares trends in language models with public opinion polls.
Read the full study, What opinions do language models reflect?
As expected, language models that form sentences by predicting sequences of words based on what others have written it should they automatically reflect popular opinion in the broadest sense. But, Santurkar says, there are two other explanations for the bias. Most of the newer models have been adjusted to human feedback data collected by companies that hire scorers to note which models are “good” or “bad”. The opinions of annotators and even those of the companies themselves can filter into the models.
For example, the study shows how the newer models have a higher than 99 percent approval rating for President Joe Biden, even though public opinion polls show a much more mixed picture. In their work, the researchers also found that some populations are underrepresented in the data: those 65 and older, Mormons, and widows and widowers, just to name a few. The authors argue that to improve credibility, language models should better reflect the nuances, complexities and narrow divisions of public opinion.
Alignment with public opinion
The team turned to Pew Research’s American Trends Panels (ATP), a benchmark survey of public opinion, to evaluate nine leading language models. The ATP has nearly 1,500 questions on a wide range of topics, ranging from science and politics to personal relationships. OpinionQA compares the distribution of the language model’s opinion on each question with that of the general US population, as well as the opinions of no fewer than 60 demographic subgroups, as charted by the ATP.
“These surveys are really useful as they are designed by experts who identify topics of public interest and carefully design questions to capture the nuances of a particular topic,” says Santurkar. “They also use multiple-choice questions, which avoid certain problems in measuring opinion with open-ended questions.”
Based on these comparisons, OpinionQA calculates three opinion alignment metrics. First, representativeness assesses how aligned a language model is with the general population, as well as with the 60 demographic sections that ATP uses. Second, orientation tabulates how well the model can reflect the opinion of a given subgroup when asked to do so. And, third, consistency predicts the strength of a model’s views across subjects and over time.
Wide variation
High level breakthroughs? All models show large variation in political and other leanings by income, age, education, etc. For the most part, Santurkar says, models trained only on the Internet tend to be skewed toward less educated, lower-income, or conservative viewpoints. Newer models, on the other hand, more refined through curated human feedback tend to be biased toward more liberal, more educated, and higher-income audiences.
“We are not saying whether it is good or bad here,” says Santurkar. “But it’s important to give visibility to both developers and users that these biases exist.”
Acknowledging that exactly matching the views of the general public could be a problematic goal in itself, OpinionQA’s developers caution that their approach is a tool to help developers assess the political biases of their models, not a point of reference of optimal results.
“The OpinionQA dataset is not a benchmark that needs to be optimized. It is useful for identifying and quantifying where and how language models are misaligned with human opinion and how models often do not adequately represent certain subgroups ,” says Santurkar. “More broadly, we hope it can spark a conversation in the field about the importance and value of better aligning language models with public opinion.”
Stanford HAI’s mission is to advance AI research, education, policy, and practice to improve the human condition. learn more.